As artificial intelligence (AI) becomes increasingly integrated into our daily lives, a recurring issue has emerged: AI hallucinations. These occur when AI models generate false or misleading information that appears plausible but is not based on real data or facts. Whether it’s in healthcare, finance, or customer service, hallucinations present both challenges and risks for those relying on AI systems.
In this article, we’ll explore what AI hallucinations are, why they happen, and what steps can be taken to mitigate their effects.
What Is an AI Hallucination?
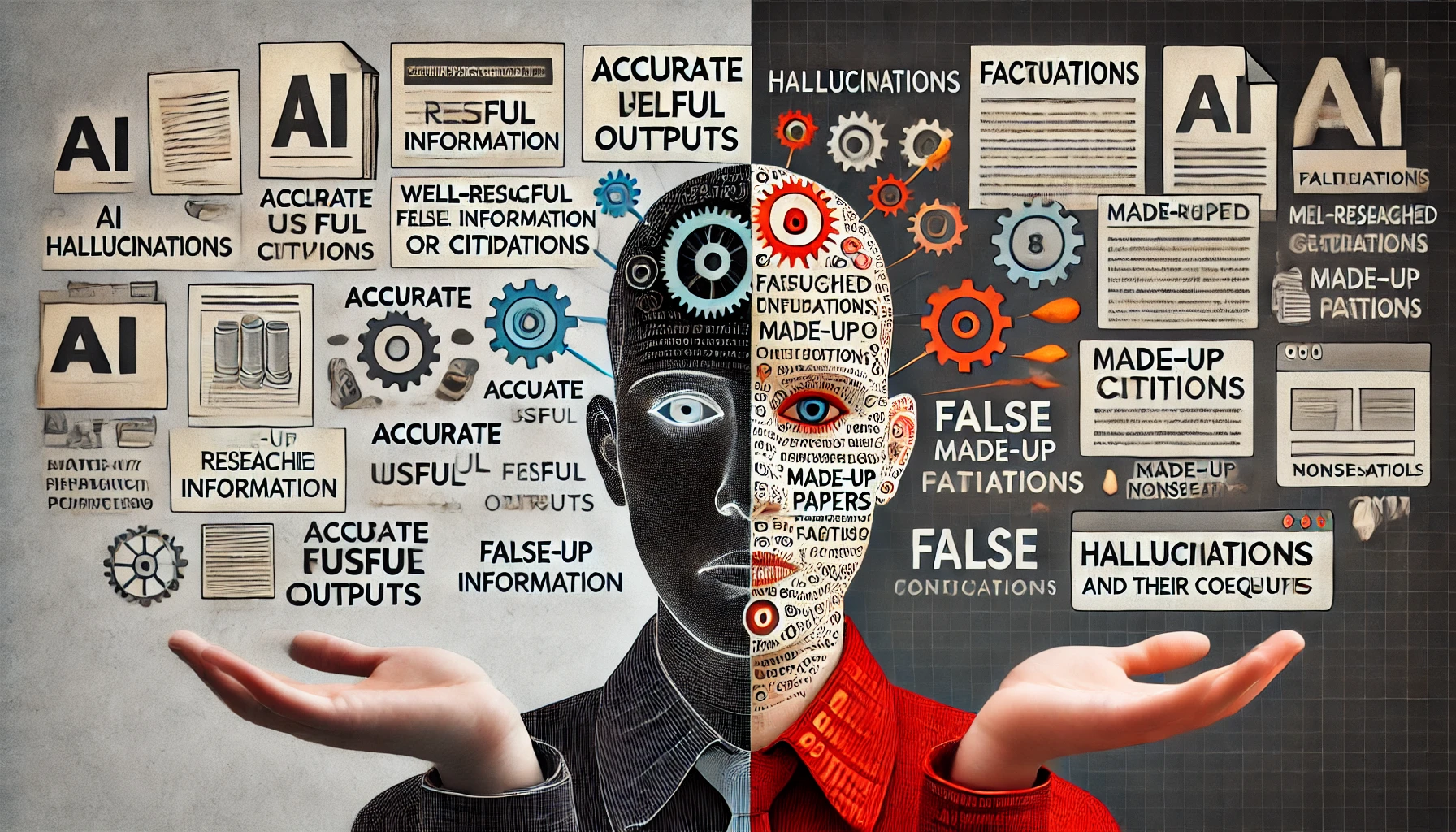
AI hallucination refers to the phenomenon where an AI system generates output that is factually incorrect or completely fabricated, despite sounding convincing. For instance, a large language model (LLM) like ChatGPT might provide a detailed explanation of a concept that does not exist, create fictional case studies, or cite non-existent research.
Why Do AI Hallucinations Happen?
Hallucinations occur due to the inherent limitations of generative AI models. These systems work by analyzing patterns in vast datasets, then predicting the most likely response to a prompt based on probabilities. While this enables impressive capabilities in natural language processing and content creation, it also introduces vulnerabilities to incorrect pattern recognition and overconfidence in fabricated details.
Several key factors contribute to AI hallucinations:
- Bias in Training Data: AI models are trained on enormous datasets, but these datasets may contain inaccuracies or biases. If the training data is skewed or incomplete, the AI might learn to generate responses that reflect these biases.
- Statistical Predictions: AI models don’t “understand” information the way humans do. They generate content based on the likelihood of certain words or phrases appearing together. This can lead to the creation of plausible-sounding falsehoods that aren’t rooted in actual knowledge.
- Complex Prompts: Some hallucinations occur when AI is faced with unexpected or ambiguous prompts. In these cases, the AI tries to “fill in the gaps” by generating content that seems reasonable but is actually incorrect.
Examples of AI Hallucination
- Healthcare: An AI model tasked with diagnosing medical conditions could mistakenly identify a benign symptom as a sign of a serious disease, leading to unnecessary interventions.
- Legal Missteps: In a widely publicized case, a lawyer used an AI tool to generate legal references, only to discover that many of the cases cited by the AI did not actually exist.
- Fabricated Citations: AI systems have been known to generate research paper citations or factual references that sound legitimate but are completely made up.
The Risks of AI Hallucinations
AI hallucinations can lead to misinformation, legal liabilities, and reputational damage. In industries like healthcare and finance, the consequences can be particularly severe. For example, if a financial AI miscalculates investment advice based on hallucinated data, users could suffer significant losses. In the legal realm, reliance on inaccurate AI outputs could lead to incorrect decisions or courtroom blunders.
How to Detect and Mitigate AI Hallucinations
- Human Oversight: One of the most effective ways to prevent hallucinations from causing harm is ensuring that AI-generated content is reviewed by a human expert before being acted upon. This is especially important in sensitive fields like healthcare, law, and finance.
- High-Quality Training Data: Ensuring that AI models are trained on diverse, well-vetted datasets can reduce the likelihood of hallucinations. This involves ongoing efforts to clean and balance training data.
- Fact-Checking Tools: Emerging tools like Nvidia’s NeMo Guardrails or Got It AI’s TruthChecker can cross-verify AI outputs against trusted sources to catch hallucinations before they reach users.
- Limiting AI Responses: By setting clear boundaries for AI outputs, such as limiting their responses to predefined templates or certain domains of knowledge, the risk of hallucinations can be minimized.
Conclusion
AI hallucinations are a complex and ongoing challenge for generative AI systems. As powerful as these models are, they still lack the ability to truly “understand” reality and can sometimes generate content that is misleading or outright false. By employing robust training data, automated verification tools, and human oversight, businesses and individuals can minimize the risks associated with AI hallucinations while continuing to benefit from the efficiency and creativity that AI offers.
FAQs
1. What causes AI hallucinations?
AI hallucinations are caused by biased training data, incorrect pattern recognition, or ambiguous prompts, leading to the generation of false or misleading content.
2. How can you prevent AI hallucinations?
Using high-quality training data, applying fact-checking tools, and maintaining human oversight are key ways to mitigate hallucinations in AI systems.
3. Can AI hallucinations be harmful?
Yes, especially in sensitive areas like healthcare or finance, where inaccurate information can lead to serious consequences, including misinformation and legal risks.
Resources for More Information
- Cloudflare – Overview of AI hallucinations, causes, and examples.
Cloudflare: What are AI Hallucinations? - IBM – Implications of AI hallucinations, prevention strategies, and use cases.
IBM: What Are AI Hallucinations? - Techopedia – Causes of AI hallucinations and tools for detecting misinformation.
Techopedia: What Is AI Hallucination?
Want to leverage AI without the risks? Schedule your free consultation with our AI experts today and discover how we can tailor solutions that are accurate, reliable, and effective for your business.